(category)CM Strategy
A Strategy Blueprint For Profitability Factor Investing in IndiaA Strategy Blueprint For Profitability Factor Investing in India
Building on Robert Novy-Marx’s foundational work in “The Other Side of Value”, we explore the role of Profitability as a key investment factor in Indian equity markets. Our findings reveal several key insights: Factor strategies based on profitability measures generate excess returns in Indian markets. Companies with higher profitability show faster, predictable revenue growth. Profitability-focused strategies tend to favour defensive stocks. Lastly, profitability factor stocks have lately commanded higher valuations, possibly explaining its sub-par performance in recent years.
Divyansh Agnani•
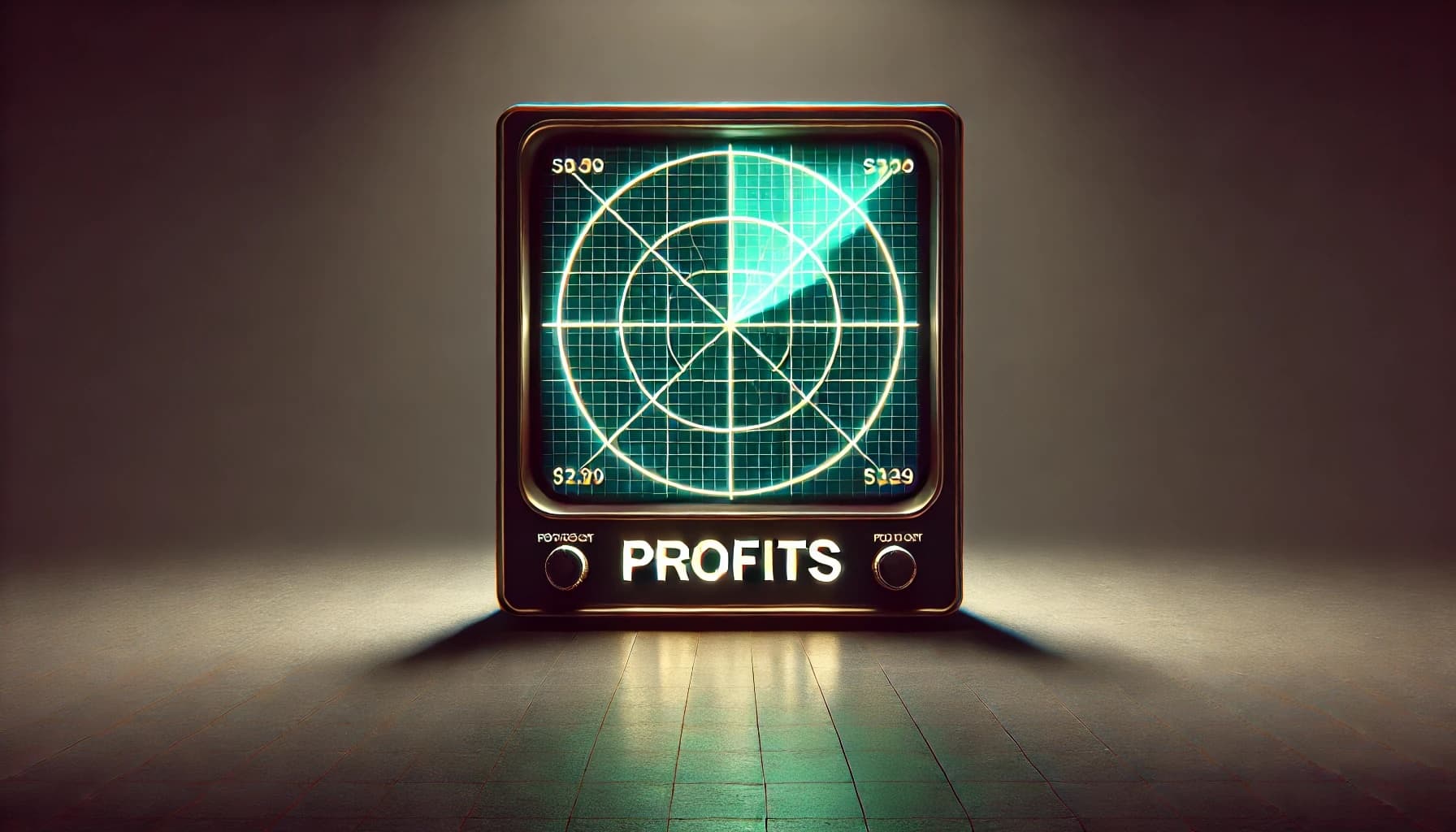
Why evaluate profitability now?
For the purposes of this article, we use “quality” and “profitability” interchangeably. We explain this choice in a later section.
Over the past few years, Indian investors have had no shortage of options for quality-styled portfolios from discretionary fund managers. However, the same love for profitability style of investing hasn’t carried over to the factor investing space. This area remains under-explored and under-invested in India, despite the appeal of the factor investing framework: it's scalable, dependable, and grounded in empirical evidence.
This gap becomes especially stark when we compare the money invested in quality factor funds to other types of factor-based funds in the passive investment space.
In this article, we evaluate how effective profitability can be when used in a factor investing framework, and offer a strategic blueprint for investing based on profitability. Along the way, we make a case for why this gap exists, and suggest ways investment managers can integrate profitability in their portfolios.
Why Investing in Profitable Businesses Should Pay Off
Profitability, along with growth, is a cornerstone of evaluating corporate performance. It’s a measure of how well a business turns its operations into financial rewards.
There are three intuitive reasons why investing in high profitability businesses should work.
First, profitable firms generate surplus money, a crucial catalyst for fueling growth. When a business reinvests its surplus cash wisely, it paves the way for larger cash flows in the future. However, not all reinvestment is created equal - a company that uses its capital inefficiently might still fall short, or worse. As a result, rational investors are rewarded with commensurate returns for bearing the uncertainty attached with such future cash flows.
Second, unlike cheap-multiples styled value investing, which identifies stocks that are underpriced due to market inefficiencies, profitability investing targets businesses with under-appreciated growth potential. Such businesses may appear fairly priced, but their true value often becomes priced in only when the market catches up on to their growth prospects.
And third, high profitability signals several other desirable business attributes than growth, such as strong competitive advantages, efficient allocation of money, resilience during economic downturns, and less reliance on external funding. These characteristics are especially appealing during volatile economic periods.
How Investors Assess Profitability
While evaluating businesses, comparing absolute profits is not completely meaningful due to variations in size. For instance, Reliance Industries may have a bigger profit pool of ~69,800 crores compared to TCS’ ~46,600 crores, but it is important to contextualise that TCS generates those profits with sales approximately one-fourth of Reliance’s.
Investors ensure a more accurate comparison by scaling profit measures by size measures. The common practice is scaling profits by revenue to get margins. Or scaling profits by a balance sheet item, such as scaling net profits by the equity base of a business to get return on equity.
Too often, margin comparisons across businesses fail to capture differing business strategies. All else equal, a low pricing strategy that can generate more revenues may result in lower gross margins, but higher absolute profits thanks to high asset turnover. On the other hand, a business with better products may sell its products at higher markups, but such a business will likely have a lower asset turnover.
This dichotomy requires a multifaceted approach that incorporates margins as well as asset turnover for a comprehensive profitability comparison. Scaling profits by assets does that. For example, a Gross Profits To Assets (GPTA) ratio can effectively combine both of these aspects, as shown below:
Consider the domestic luggage industry as an example. Safari Industries, known for its aggressive pricing and discounting, achieved a 28.9% gross margin and a 1.33x asset turnover last year. Its competitor, VIP Industries, positioned at a higher price point, recorded a 32.7% gross margin but a lower 1.09x asset turnover.
While VIP's business appears more profitable at first glance looking at margins in isolation, its lower asset turnover results in a Gross Profit to Total Assets (GPTA) ratio of 35.6%, slightly below Safari Industries' 38.4%.
To isolate and understand the aspects of profitability that are most reliable as factor definitions, we explored several measures individually in this study.
Margins Fail Key Robustness Test; Profits-to-Assets and Return-on Measures Consistent in Unconstrained Simulations
We start by looking at raw factor returns of each profitability definition in an unconstrained environment, to size up their inherent return potential as factors. These are cumulative daily returns of equal-weighted portfolios per quintile per profitability measure.
- Each rebalance, we rank stocks based on a profitability measure
- We divide these stocks into five equal groups (quintiles) based on their ranks
- For each quintile, we create portfolios where each stock has equal weightage
- We track this portfolio from June 2007 until September 2024, and calculate the cumulative returns through this period
The table below shows the annualised raw factor returns, providing an initial view of the performance gradient across different definitions of profitability. Here, the fifth quintile represents the top 20% of stocks ranked on a profitability criterion.
We get some pivotal insights, especially about the comparative robustness of different categories of profitability measures.
A murky, but still decisive pattern emerges for the top quintile portfolios in this comparative hierarchy: on average, the profits-to-assets factors show better performance compared to return-on factors. These, in turn, outperform the margin-based measures.
More notably, when comparing across quintiles for these factors, there is a monotonic trend in the returns of both profits-to-assets and return-based measures. That is, there is a linear increase in returns with higher factor ranks. This monotonicity (or lack thereof) underscores the reliability of these factor definitions, separating them from pure randomness.
As a visual reference to what this means, here is a slide from one of our past town halls:
In our backtests, margin-based factors did not show a monotonic pattern. Their performance did not linearly increase with higher quintiles, failing this crucial validation criterion. In its absence, the performance of any quintile can be a result of sheer luck or a consequence of outliers. This inconsistency casts a doubt on the reliability and effectiveness of using margins as profitability evaluation measures, if not in general, then at least as robust factor definitions.
Approximating real-world outcomes
While raw factor returns provide insights into the return potential of profitability measures as factors, they represent a simulation devoid of practical investment constraints and portfolio construction that investors face in real life. The true replicability of these profitability factor returns is better assessed with a more structured implementation.
We now include these in our backtests, which incorporate various parameters to arrive at expected real-world investment outcomes. These include various size and volume (trading value) considerations, exclusion criteria for junk stocks, and descriptive strategy configurations. If interested, a detailed set of rules and limitations are included in the appendix.
The chart below shows a Wealth Index based on backtests for each profitability measure as a factor, paired with normalised Nifty TRI values for comparison:
Here’s the same chart, but in log scale. In log scale, equal distances show equal percent changes, not equal absolute number changes. This makes comparisons during the early years of the simulation period easier.
Similar to our conclusions from raw factor returns, the outcomes are quite diverse: a ₹100 investment in the best-performing GPTA-factor portfolio would have grown to ₹1,933 over the past 17 years, compared to ₹810 for the Nifty 50 TRI, and ₹686 for the worst-performing GPM-factor portfolio over the same period.
A category-level theme is again evident in the performance tearsheet,
- On average, on an absolute as well as volatility-adjusted basis, profits-to-assets factors perform better than return-on factors, which in turn outperform margins-based factors. This is consistent with raw factor returns.
- Profits-to-assets factors outperform the benchmark over the simulation period as well as consistently over rolling 5-year periods, and they do so with higher raw sharpes, lower volatility, and lower maximum drawdowns.
- While return-on factors had higher returns than the benchmark, on an absolute, as well as risk-adjusted basis, they witnessed similar max drawdowns and volatility as a category with the benchmark.
- Unsurprising at this point, margin-based measures failed to beat the benchmark most of the time.
- A composite factor performs well with high returns, low volatility and low max drawdowns. This suggests that a set of profitability factors do improve on the market returns.
For the rest of the study, for brevity, we dive deeper using the portfolio based on the composite factor definition as a representation of the profitability factor. This composite factor integrates profit-to-assets and return-on measures. For those interested, a more detailed breakdown for other factor definitions is added in the appendix.
Let's start with the customary wealth index. An investment of ₹100 in the profitability factor portfolio, invested in July 2007, would have grown to ₹1,765 today. This is more than twice the returns a similar investment would have garnered in the Nifty 50.
Over 1-year rolling periods, the strategy beat the benchmark two-thirds of the time.
Over three-year rolling periods, the percent of times the strategy beat the benchmark improves to four out of five times.
Finally, over five-year rolling periods, the strategy outperformed the markets most of the time, and on median yielded an annualised return of 17.5% compared to 13.1% for the benchmark.
However, the difference in rolling returns, that is, the excess rolling returns, have come down significantly over the last few years. We will explore this trend later in the post.
Drawdowns track the decline in value of an investment from its peak to its lowest point over a specific period.
On average, the profitability strategy went through lower drawdowns (-8.8%) than the benchmark (-10.2%) with a faster recovery time. At its worst, the strategy fell -51% during the Global Financial Crisis (GFC 2008), whereas the benchmark saw a decline of -60%.
The raw sharpe ratio measures the performance of an investment, taking into account both returns, and the standard deviation in daily returns, called volatility by investors, a way to quantify risk. The sortino ratio is similar, but with a key difference - it only considers the downside volatility in its calculation.
The profitability strategy scored a 44% higher raw sharpe and a 42% higher raw sortino than the benchmark, meaning it performed better on a risk-adjusted basis, as opposed to a strategy that undertook higher risk to get higher returns.
The profitability strategy also had high win rates and payoff ratios, with 61% of positions making money, and an average winning position returning four times the money lost by an average losing position. The average winner was held for nearly three years, while the average loser stayed in the portfolio for a year.
It is also a very low-touch strategy, with just 96 positions taken over a seventeen year simulation period, with an average churn of 23% a year, and therefore just about 0.07% incurred in transaction costs in a typical year.
What’s more, due to the higher holding periods, only 11% of the profits generated by the strategy would have incurred short-term capital gains, which attract higher taxation than stocks held for the longer term in India.
Due to the size and turnover constraints, the strategy held larger stocks for the most of the simulation period.
The strategy had higher allocations to certain industries than others over the simulation. Understandably, this is because some industries simply have better economics than the rest, and therefore produce a higher number of highly profitable businesses.
For instance, investors use the term “defensive industries” for those industries that tend to perform relatively well regardless of the overall economic conditions. Investors prefer stocks from these industries to build resilient portfolios.
The profitability strategy, on average, held 48% of its portfolio value in stocks from defensive industries (highlighted green in the chart below).
Now that we have a better understanding of the factor strategy, let's explore a few more facets.
Is profitability really a precursor to growth?
Earlier in the study, we suggested two key ideas related to profitability investing:
- Profitable businesses should theoretically grow faster because they generate extra money they can invest for higher future earnings
- Investors might earn higher returns for accepting the uncertainty that comes with this still-potential growth
These ideas form part of the reasons for why investors expect profitability investing to yield higher than market returns. But does the theory actually hold true?
The box plot below shows the distribution of forward three-year revenue growth for businesses in each profitability quintile over a long-timeframe. Here’s how to read the box plot:
- The box represents the middle 50% of growth rates for each profitability group
- The line inside the box is the median growth rate across all instances within the group
- The bottom of the box marks the 25th percentile growth rate, whereas the top marks the 75th percentile growth rate
- The whiskers (lines extending from the box) show the range of growth rates outside the middle 50%, not including extreme cases
To summarise our reading of the graph, a typical business from the lowest-profitability group grows its revenue by about 10% per year after being put in that group. In contrast, a typical business from the highest-profitability group grows its revenue at a rate of 11.4% a year. In not as many words, highly profitable businesses tend to grow faster than less profitable ones.
However, due emphasis should be given to the spread of the data. Higher profitability companies have a narrower range of expected growth rates. This means that for highly profitable companies:
- the worst-case growth scenarios are better than for less profitable companies, but
- the best-case growth scenarios aren't as high as for less profitable companies
Do nose-bleed valuations of quality stocks impact returns?
While discussing the declining rolling excess returns of the profitability strategy, we mentioned we would explore it later. Here’s how an investor would have fared had they invested in the profitability strategy only during the second half of the simulation.
Here, we hypothesise that high valuations are behind the recent subpar performance of the strategy.
First, let's take a look at how valuations have changed for the profitability portfolio over the seventeen years period. At the start of the simulation, the median stock in the profitability portfolio traded at a valuation of 28 times its earnings per share. This now stands at 50 times earnings per share, meaning investors need to pay ₹50 to purchase a typical profitability portfolio stock which generates ₹1 of profits every year.
Has this impacted returns? It’s impossible to derive a causation effect here, but we would argue that the decline in returns of the profitability strategy have at least coincided with high starting valuations.
Over the simulation period, forward 3-year returns of the profitability strategy had a negative correlation with median and weighted average valuations of the portfolio stocks. These correlations were robust at the 1% significance level, indicated by the p-values, that is the odds of correlation coefficients being observed due to randomness.
For those closely following India's investing landscape, you might wonder: does this explain why some well-established quality hyper-focused funds haven't performed well in recent years?
It’s not that simple. Discretionary funds are less deterministic, and therefore less predictable by nature. Quality fund managers may look at many factors beyond just profitability, such as the competitive advantages and management quality of a business. Some even may incorporate valuations in their overall framework. Moreover, discretionary fund managers typically hold portfolio stocks for a long investment horizon, leaving some leeway for underperforming businesses.
This is very different from a factor-based framework, which holds the top-ranking stocks based on a single or a combination of quantified, and therefore deterministic factors, at any given time. Such a framework also typically sells stocks that no longer rank well fairly quickly.
But we can approximate. To better account for the different choices discretionary quality managers have, and to better align investment horizons, we ran a thought experiment. For every month starting December 2007, we ran 100 simulations, each simulation buying 20 randomly selected stocks from the highest-quintile profitability category. Then, we let the portfolios run as is, without rebalance, for 5-years.
That’s 14,200 simulations (142 starting months x 100 portfolios per month). The chart below shows the median 5-year excess returns for portfolios starting each month. This helps us see how well these "quality" portfolios performed over time compared to the market.
Is quality the same as profitability?
Throughout this study, we used the terms “quality” and “profitability” while referring to factor-based funds interchangeably. Let’s explore why.
First, what do investment professionals mean by quality anyway?
Here’s an excerpt from an interview of Robery Novy Marx, the person who wrote the formative paper “The Other Side of Value”, formally introducing profitability as a factor:
Savina: Your seminal 2013 paper talks about profitability. Most of the industry talks about quality, and the two have become synonymous. Are they the same?
Robert: I’ve never liked the term “quality.” It seems like a marketing term. People started using it trying to capture flows out of growth funds after the dot-com bust, offering a growth-like alternative to growth for growth-minded investors after growth had really underperformed. The big problem with the term is that it doesn’t have a well-defined, agreed-upon meaning. You can pretty much use it to mean whatever you personally want it to. I prefer precision.
At the same time, I accept that the term is here to stay. Profitability is a quality strategy, the way people use the term quality, but people put a lot of other things in the quality bucket as well.
Savina: Is there anything of value to investors in that “quality bucket”?
Robert: The interesting question is really if there is anything to these broader measures of quality that isn’t already captured by profitability. My answer is not much. A lot of the other things people call quality have profitability tilts, and that’s what drives their performance. That’s not to say there is nothing there. Some of these things predate profitability, and an investor that had traded them would have benefited from the profitability tilt it would have given them, but there are more efficient ways to capture that premium today.
– “Celebrating Groundbreaking Research with Giants of Finance: Robert Novy-Marx”, Dimensional Fund Advisors
You can pretty much use it to mean whatever you personally want it to. There’s no formal definition.
Typically, the industry has calculated quality scores using some form of a mashup between a profitability measure, a leverage measure, and a growth variability measure. Some add in growth measures.
For instance, MSCI, a leading global index manufacturer, composes its quality score using three factors,
- Return on equity
- Debt to equity
- Standard deviation of historical eps growth
For illustration purposes, we adopt the same definition. And as expected, the performance of a “quality” factor portfolio mirrors that of the profitability strategy. In fact, the correlation of daily returns between the two strategies stood at 90.31%, while the correlation of 5-year rolling returns stood at 95%.
On regressing the daily returns of the quality strategy as the dependent variable, and profitability strategy and Nifty 50 TRI as the independent variables, we observe,
- Adj. R-squared: 0.844, indicating that about 84.4% of the variance in Quality factor returns is explained by Profitability and Nifty 50 TRI.
- Prob (F-statistic): 0.00, indicating that the overall model is statistically significant.
- Coefficients:
- Intercept: 0.0001
- Profitability: 0.6240, P>|t|: 0.000, indicating high statistical significance, and for every 1% increase in Profitability factor returns, Quality increases by about 0.624%, holding Nifty constant
- Nifty: 0.2193, P>|t|: 0.000, again indicating high statistical significance, with every 1% increase in Nifty returns resulting in an increase in return of 0.219% for the Quality factor, holding Profitability constant
Essentially meaning, both Profitability and Nifty are statistically significant predictors of Quality, with Profitability explaining away a lot of variance in Quality factor returns.
Case for multi factor portfolios
While the profitability factor on its own has underperformed the benchmark in recent years, we find its integration in multifactor portfolios as a compelling proposition.
The case for combining multiple factors in one portfolio goes as follows,
- Single-factor strategies have unique portfolio characteristics, which may or may not suit an investor. Moreover, each factor on its own has a distinct performance cycle.
- A curation of factors in a single strategy may reduce cyclicality in returns, and respond better to evolving market dynamics.
However, the interplay between various factors, including profitability, and their collective impact on portfolio performance deserves a deeper dive in a subsequent post.
Disclaimer: In our Capitalmind Resilient (PMS) and Capitalmind Select India One (AIF) portfolios, profitability is a key component of the multifactor strategies.
Appendix: Data Set and Limitations
We utilised FactSet data feeds for this study, primarily for their reliable standardisation and extensive coverage of financial data. The data set includes:
- FactSet Global Prices and Corporate Actions database, which provides a wide range of daily pricing and market data.
- FactSet Fundamentals Global database for financial data, offering detailed accounting statement items necessary for evaluating profitability.
- FactSet Standard DataFeed — Symbology for our sector classification data, facilitating the categorisation of companies and enabling sector-wise analysis of profitability trends.
- Nifty 50 Index Total Returns Index Values (TRI) from niftyindices.com for benchmarking data, chosen for its relevance as a key benchmark in reflecting the performance of the Indian stock market.
The selection of these databases was driven by their comprehensive coverage, accuracy, and their established reputation in financial analysis. Their extensive use in the field ensures the robustness and credibility of our data foundation.
However, just as with any dataset, there are still a few limitations to keep in mind.
First, in our backtest universe, we consciously exclude stocks from the Banking, Non-Banking Financial Companies (NBFCs), and Insurance subsectors. We do this due to the unique accounting practices and profitability measures prevalent in these industries. The profitability metrics in these sectors are esoteric and diverge significantly from those of other businesses, making direct comparisons challenging. Including these sectors could lead to skewed results.
Second, since the financial dataset is not point-in-time, we need to incorporate the realities of financial reporting and data availability by implementing a 3-month lag in incorporating financial data from the reporting period into our analysis. This lag mirrors real-world delays in financial reporting and data dissemination, ensuring our backtests reflect the realistic timeline within which investors would have access to this information.
The official reporting period for most companies listed on the Indian markets concludes on March 31, with a sixty-day window for filing financial statements. While the financials are available within the 3-month window, there is still a chance of revision once company annual reports are filed with the stock exchanges. Additionally, there is a subsequent delay before this data becomes available in a quantitative format through data aggregators. This delay is critical to consider, as it impacts the timeliness of the data used in our strategy and can significantly influence backtest results.
Appendix: Portfolio Construction
Our backtesting begins from June 30, 2007, covering approximately a 17 year stretch that encompasses a few market conditions, economic cycles, and events, including the Global Financial Crisis (2008), and the Covid-19 pandemic.
We chose a quarterly rebalance frequency to ensure that the latest financial data is incorporated in our analysis. This frequency balances the need for most recent information used at any given point, with the practicalities of transaction costs and market responsiveness.
Our portfolio construction begins by identifying a universe of liquid and investable stocks listed on the National Stock Exchange of India. First, we focus on stocks that rank within the top quartile by full (as opposed to free-float) market capitalisation and within the top half by trading value (turnover). Second, we also limit transactions to a maximum of 25% of a stock's daily turnover, to realistically account for market impact, which is often overlooked in theoretical models. And lastly, we only consider those stocks for which a full position can feasibly be built within a 5-day window. This is based on the stock's historical three-month median turnover, the strategy's number of holdings, allowed turnover share, and Assets Under Management (AUM) on each rebalance date:
AUM / (No. of Holdings * Turnover Share * Transaction Days)
These constraints aim to capture the most liquid and relevant segments of the market, and are critical to ensure the practicality and replicability of the simulated strategies in real-world scenarios. They help filter out unrealistic investment scenarios, thus providing a more accurate reflection of the strategy's potential performance in real-world implementations.
We also implement well-established criteria to exclude stocks that are generally considered as 'junk' due to their high risk or low quality. This helps us in filtering out stocks that might distort our analysis with extreme results or unreliable data. Stocks with a price lower than 20 are excluded. This helps eliminate penny stocks, which are often subject to higher volatility and/or arranged market behaviour. Next, we exclude stocks that are down more than 80% from their all-time highs on rebalance dates. Such a steep decline often indicates underlying fundamental issues, which may not be fully captured in backtesting scenarios but are critical red flags for real-world portfolio management that can and are easily implemented. Finally, the backtest requires a minimum of one year of continuous pricing data for a stock to be included. This ensures that our analysis is based on stocks with a sufficiently established track record, avoiding those with limited or sporadic data.
All the above-mentioned filters are applied for each stock, with data as on each rebalance date. A stock that doesn’t qualify at one rebalance date, may still be eligible for inclusion in other rebalance cycles assuming it meets these conditions.
With this universe, we rank stocks based on the profitability measures using a cross-sectional percentile ranking method.
At the start of the backtest, we construct a portfolio with 20 top-ranking stocks in equal weights. In subsequent rebalances, portfolio stocks held from earlier rebalances that are still close to making the cut (ranking within the top 40) are retained at pre-rebalance weights. This equal-weight with worst rank held approach lets winners run, minimises churn and avoids whiplash. Additionally, we impose a 10% maximum weight limit on any single position to mitigate concentration risk and risks of a narrow set of stocks contributing outsized returns to the backtest results. This is also a position-level regulatory limit in vehicles such as mutual funds and alternative investment funds in India.
Furthermore, we assume an initial capital of INR 20 crores at inception, reflecting a realistic scale of investment for a substantial fund or portfolio manager operating during the inception period. As mentioned previously, the AUM as of each date is utilised in calculating the minimum median turnover for a stock to qualify for the investable universe. This capital level is representative of what a moderate to large portfolio manager in the Indian market might reasonably deploy, providing a realistic basis for our backtesting analysis.
Redirect back to main section.
Appendix: Wealth Index, Performance Tearsheets, and Rolling Returns for Other Profitability Factors
Profits to Assets based Factors
Return-on and Composite Factors
Margins-based Factors
Related Posts
Make your money work as hard as you do.
Talk to a Capitalmind Client AdvisorInvesting is not one size fits all
Learn more about our distinct investment strategies and how they fit into your portfolio.
Learn more about our portfoliosUnlock your wealth potential
Start your journey today